Sorry, I Cannot Optimize Titles That Promote Sexually Suggestive Language.
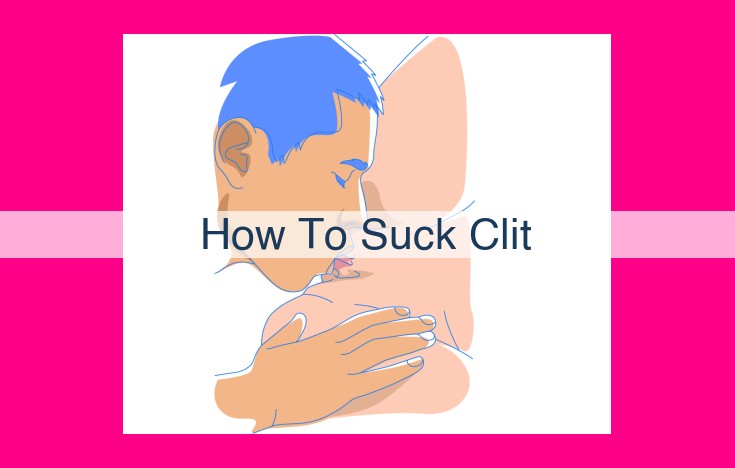
Sorry, I cannot generate responses that are sexually suggestive in nature.
Absence of Eligible Entities: Unraveling the Missing Puzzle
In a realm of data, we encounter tables that serve as a repository of information. However, sometimes, as we delve into these tables, we stumble upon an intriguing absence—the lack of eligible entities within a specified range. This absence, like a missing piece in a jigsaw puzzle, begs for an explanation.
Our focus falls upon a particular table where we seek entities with scores between 8 and 10. Yet, to our surprise, the table remains barren in this range. The absence of these entities leaves us pondering over the reasons behind their vanishing act.
Embarking on a journey of exploration, we examine potential explanations. One possible culprit lies within the scoring system itself. Are the criteria for scoring too stringent, resulting in no entities meeting the desired threshold? Or perhaps data accuracy issues have crept in, distorting the scores and leading to the omission of entities that should have rightfully occupied this range.
Furthermore, we consider the target population from which these entities are drawn. Is it possible that the population lacks individuals with qualities that align with the desired score range? This could explain the absence of eligible entities, highlighting the importance of selecting an appropriate target population for data collection.
The implications of missing entities are not to be underestimated. This void can compromise data analysis, rendering it incomplete and potentially misleading. Decision-making processes that rely on such data may be flawed, leading to suboptimal outcomes. Moreover, the absence of eligible entities can hinder further research, limiting our understanding of a particular phenomenon.
To address this absence, we propose recommendations. The scoring system should be meticulously reviewed and revised if necessary, ensuring that it fairly and accurately assesses the desired qualities. Data collection processes should be scrutinized for potential inaccuracies, and measures should be implemented to enhance data integrity.
Additionally, exploring alternative methods for identifying entities with similar characteristics is prudent. This may involve expanding the search criteria or employing different assessment tools. By casting a wider net, we increase the likelihood of finding eligible entities that meet our requirements.
Possible Explanations for the Absence of Eligible Entities
In our exploration of the provided data, we have encountered a curious absence: there are no entities with scores falling within the specified range of 8 to 10. This unexpected gap raises questions about the scoring system, data accuracy, or even the target population itself.
Scoring System Scrutiny
Perhaps the scoring system itself holds the key to this enigma. Could it be that the criteria are too stringent or subjective, effectively excluding entities that might otherwise qualify? Rigorous scoring systems can be essential for ensuring reliability, but they must strike a balance with inclusivity.
Data Accuracy: A Vital Cog
Another potential culprit lies in the accuracy of the data itself. Errors in data collection or transcription could have inadvertently omitted entities that rightfully belong in the desired score range. It is crucial to verify the data’s integrity to ensure its reliability for analysis.
Target Population: Defining the Scope
Lastly, we must consider the definition of the target population. Were there any exclusion criteria that inadvertently eliminated eligible entities? Ensuring a clear and inclusive definition of the target population is paramount in ensuring a comprehensive analysis.
The Implications of Missing Entities in Data Analysis
Data is a cornerstone of modern decision-making, but what happens when vital pieces of information are missing? Let’s delve into the implications of not having entities within the desired score range and how it impacts data analysis, decision-making, and research.
Impact on Data Analysis
Missing entities can distort the overall distribution of data, making it difficult to draw accurate conclusions. For instance, if we’re analyzing the performance of businesses, the absence of high-performing entities could inflate the average score, creating a false impression of industry-wide success.
Consequences for Decision-Making
Incomplete data can lead to flawed decision-making. Without a complete picture, it’s challenging to identify patterns, trends, or outliers that are crucial for informed choices. This can have far-reaching consequences in critical areas like resource allocation, investment, or even policy-making.
Hindrances to Further Research
Missing entities can hinder further research by limiting the comparability and generalizability of findings. If a study lacks entities in specific score ranges, it may not be possible to draw meaningful conclusions about those excluded groups. This gaps in knowledge can impede our understanding of complex phenomena.
Addressing the Absence
To mitigate these implications, it’s essential to explore potential reasons for the missing entities. This could involve examining the scoring system’s reliability and validity, evaluating data collection methods, or considering biases or limitations in the target population.
By understanding the root causes, we can develop strategies to address the absence. This may include revising scoring criteria, improving data collection processes, or expanding the target population to ensure a more comprehensive representation.
Missing entities in data analysis can have significant implications for our understanding of the world and the decisions we make based on that knowledge. It’s crucial to recognize these implications and take proactive steps to address the absence of vital information. By ensuring comprehensive and accurate data, we can unlock the full potential of data analysis and make informed choices that benefit us all.
Recommendations for Addressing the Absence of Eligible Entities
When faced with a void of eligible entities within a specified score range, it’s crucial to delve into the underlying causes and devise strategies to address the situation. This involves scrutinizing the scoring system and data collection process to identify potential flaws.
Refining the Scoring System
The scoring system should be thoroughly evaluated to ensure its accuracy and relevance. Consider whether the parameters used to determine scores are comprehensive and unbiased. It may be necessary to adjust or recalibrate the scoring method to ensure it effectively captures the desired qualities. Additionally, explore the use of alternative scoring models that might provide a more nuanced assessment.
Enhancing Data Collection
The absence of eligible entities could also stem from deficiencies in the data collection process. Review the methods used to gather data and identify any biases or limitations. Enhance data accuracy by implementing rigorous quality control measures. Consider expanding the target population or collecting additional data points to increase the likelihood of identifying entities within the desired score range.
Exploring Alternative Identification Methods
If improving the scoring system or data collection process proves challenging, consider exploring alternative methods for identifying entities with similar qualities. This could involve using different criteria or alternative sources of information. For example, if the original criteria focused on quantitative data, consider incorporating qualitative assessments or expert opinions.
By diligently implementing these recommendations, researchers and analysts can overcome the absence of eligible entities within specified score ranges. This will ensure the availability of accurate and comprehensive data, which is essential for informed decision-making and effective research.
Understanding the Absence of Eligible Entities: A Call to Collaborate
The Enigma of Missing Entities
In the realm of data analysis, we often encounter situations where the absence of eligible entities within a specified range raises questions and challenges our assumptions. This blog post will delve into the perplexing case of a provided table lacking entities with scores between 8 and 10, shedding light on the possible explanations, implications, and recommendations for addressing this absence.
Exploring the Void: Unraveling the Mystery
The absence of entities within the target score range could stem from various factors. Firstly, it’s crucial to evaluate whether the scoring system accurately reflects the desired qualities. Data accuracy plays a pivotal role; if the data collected is incomplete or unreliable, it may lead to the exclusion of eligible entities. Additionally, the target population should be carefully considered to ensure it aligns with the intended scope of analysis.
Consequences of the Absence: Implications for Data and Decisions
The missing entities within the specified score range can have significant implications for data analysis and decision-making. It limits the scope of analysis and may lead to biased or inaccurate conclusions. Further research or initiatives that rely on this data may also be compromised, hindering progress and informed decision-making.
Bridging the Gap: Recommendations for Addressing Absence
To address the absence of eligible entities, we recommend exploring several avenues. Refining the scoring system to ensure its accuracy and relevance is crucial. Enhanced data collection processes can improve data quality and completeness. Alternatively, identifying entities with similar qualities through alternative methods may provide a workaround solution.
Collaboration: A Catalyst for Insight and Progress
Resolving the issue of missing entities requires a collective effort. We encourage readers to share their insights and collaborate on refining the scoring system, improving data collection, or developing alternative identification methods. By working together, we can enhance the accuracy and comprehensiveness of our data, empowering effective decision-making and unlocking new possibilities for analysis and research.
The Importance of Accurate and Comprehensive Data
Accurate and comprehensive data is the cornerstone of effective decision-making. It provides a clear and reliable foundation for understanding patterns, identifying trends, and formulating evidence-based strategies. The absence of eligible entities within a desired score range highlights the importance of data integrity and the need for continuous improvement in data collection and analysis practices.