Ultimate Guide To Movie Recommendations: Unlocking Personalized Movie Experiences
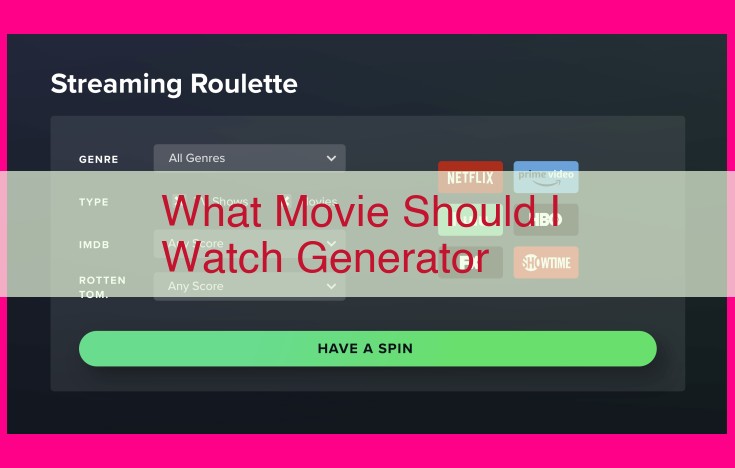
Explore the world of movie recommendations with our comprehensive guide! Discover the core principles, associated entities, and algorithms that drive personalized suggestions. Discuss ethical considerations and future trends, empowering you to make informed choices and enhance your movie-watching journey.
Core Entities: Exploring the Foundation of Movie Recommendations
Let’s dive into the fascinating world of movie recommendation systems. Imagine scrolling through a seemingly endless list of movies on your favorite streaming service. How do these platforms know which ones you’ll love? It’s all thanks to a complex system that leverages core entities to predict your movie preferences.
One of these core entities is movie genres. Just think about it: when you watch a thrilling action movie, you’re more likely to enjoy similar ones. Recommendation systems recognize this pattern and use it to suggest movies that align with your genre preferences. Subgenres play a key role too, helping systems understand your specific tastes within broader genres.
Another crucial core entity is movie ratings and reviews. When you rate or review a movie, you’re providing valuable feedback that shapes the recommendations you receive. Systems analyze these ratings and reviews to identify patterns and trends, helping them predict which movies you’ll most likely enjoy.
Associated Entities: Partners in the Movie Recommendation Ecosystem
In the cinematic tapestry of movie recommendations, streaming services emerge as indispensable platforms, weaving together a seamless web of entertainment. Like celestial bodies guiding lost voyagers, they illuminate the vast expanse of cinematic choices, offering a curated universe of personalized recommendations that cater to each viewer’s unique tastes.
Movie databases, the unsung heroes of recommendation systems, stand as veritable repositories of knowledge, providing the raw material that fuels personalized experiences. Within their vaults lie a treasure trove of information: movie genres, subgenres, actors, directors, and more. These databases are the foundation upon which recommendation algorithms build their intricate webs, mapping connections between movies and viewers.
Streaming services and movie databases form a symbiotic relationship, each contributing its unique strengths to the seamless flow of movie recommendations. Together, they orchestrate a symphony of entertainment, delivering cinematic experiences that resonate with the hearts and minds of viewers, one recommendation at a time.
Behind the Scenes: Algorithms and Data at the Heart of Recommendations
Dive into the fascinating world of movie recommendation systems, where algorithms and data orchestrate a seamless journey of cinematic discovery. These systems analyze our preferences and behavior to reveal hidden gems and tailor recommendations just for us.
Unveiling the Algorithms
Just like the invisible hand guiding our movie choices, algorithms are the driving force behind recommendation systems. Two primary types stand out:
-
Collaborative Filtering: This algorithm weaves together the wisdom of the crowd. It examines ratings and interactions from other users with similar tastes to predict movies we might enjoy.
-
Content-Based Filtering: As its name suggests, this algorithm delves into the content of movies themselves. It analyzes movie descriptions, genres, and even specific scenes to identify films that match our preferences.
Treasure Trove of Data
Recommendation systems feast on a wealth of data to make informed recommendations. These data sources include:
-
User Interactions: Our ratings, watch history, and browsing behavior provide a detailed portrait of our movie preferences.
-
Movie Metadata: Movie titles, descriptions, genres, release dates, and even cast and crew information offer valuable insights into movie characteristics.
-
External Sources: Reviews, social media buzz, and external databases enrich the system’s understanding of movie popularity and reception.
Stitching It All Together
Algorithms seamlessly blend these data sources to create personalized recommendations. Collaborative filtering harnesses the wisdom of our peers, while content-based filtering mines the rich tapestry of movie content. Together, they present us with a curated selection of movies that align with our tastes.
Behind the scenes of movie recommendation systems, algorithms and data work in harmony, leading us on a cinematic adventure. By understanding the algorithms at play and the data that fuels them, we appreciate the intricate mechanisms that bring us the perfect movie matches, time and time again.
Ethical Considerations: Responsibility and Fairness in Movie Recommendations
Movie recommendation systems have become an integral part of our streaming experience, helping us discover new and engaging films that align with our tastes. However, with great power comes great responsibility, and it’s crucial to address the ethical implications that arise with these systems.
One of the primary concerns is the creation of filter bubbles, where we are only shown content that matches our existing preferences. While this can initially provide a personalized experience, it can also lead to a narrow and potentially biased view of the world. Recommendation systems should strive for diversity and challenge users to explore films that may lie outside their comfort zone, broadening their cinematic horizons.
Another ethical concern is algorithmic bias, which arises when recommendation algorithms amplify biases present in the training data. This can lead to systemic unfairness, where certain groups of users consistently receive recommendations that are less relevant or even discriminatory. It’s essential that these algorithms are developed with fairness and transparency in mind, with measures in place to mitigate bias and promote inclusivity.
Principles and Practices for Ensuring Fairness and Transparency
To ensure that movie recommendation systems are fair and responsible, several principles and practices should be adopted:
- Transparency: Openness about the algorithms and data used to generate recommendations fosters trust and allows users to understand how their choices influence the content they see.
- Diversity: Algorithms should promote a wide range of films, reflecting diverse perspectives and experiences, to avoid the formation of filter bubbles.
- Accountability: Developers and providers of recommendation systems must be held accountable for the fairness and transparency of their algorithms.
- Feedback Mechanisms: Users should be given the ability to provide feedback on the recommendations they receive, allowing them to shape the system and ensure their preferences are respected.
By incorporating these principles into the design and implementation of movie recommendation systems, we can create a future where technology empowers our cinematic experiences while upholding ethical values of fairness and inclusivity.
Future Trends: Innovating and Evolving Movie Recommendations
The world of movie recommendations is on the cusp of exciting advancements driven by emerging technologies. One such technology is AI (Artificial Intelligence), which has the potential to revolutionize the way movies are recommended to users.
AI-powered recommendation systems can analyze vast amounts of data to identify intricate user preferences and patterns. By leveraging machine learning algorithms, these systems can personalize recommendations based on a user’s past viewing history, likes, dislikes, and even their current mood. This enhanced personalization promises a more seamless and tailored experience for movie enthusiasts.
Another transformative technology is machine learning. Machine learning algorithms can be trained on vast datasets of movies and user data to learn complex relationships and correlations. This enables recommendation systems to not only suggest movies that a user may enjoy but also provide reasoned explanations for their choices. Such transparency fosters trust and deepens user engagement with the recommendation process.
Future-forward movie recommendation systems will integrate these technologies to create a more intuitive and serendipitous experience. By leveraging AI and machine learning, these systems will continuously adapt and refine their recommendations, exposing users to a wider range of movies that align with their evolving tastes.
In addition, the rise of streaming services has fueled the demand for real-time recommendations. These services can track what a user is watching and suggest compatible movies to watch next. Such contextual recommendations enhance the viewing experience and make it effortless for users to discover new content.
The future of movie recommendations is one where technology serves as a seamless facilitator, connecting users with the movies they truly want to watch. AI and machine learning will empower users with personalized, transparent, and ever-evolving recommendations, transforming the movie-watching experience into a tailor-made journey of cinematic delight.