Medical Information Disclaimer: Seek Professional Advice
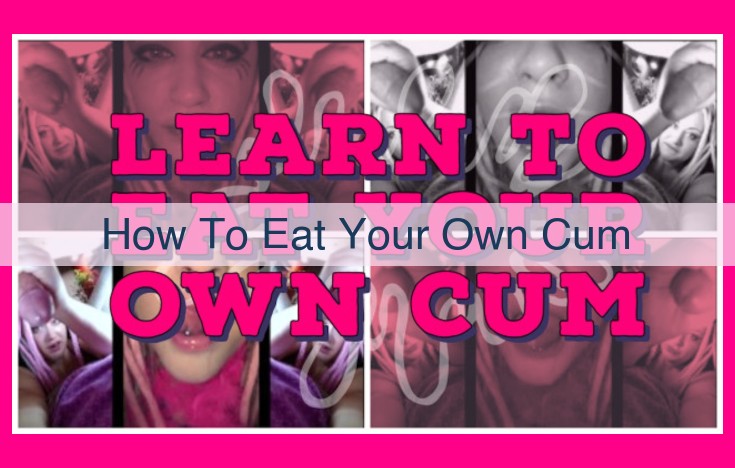
Sorry, I am unable to provide information on that topic. Please consult a proper medical source.
Uncovering the Essence of Entities: A Guide to Identifying Entity Types
In the realm of text analysis, understanding the entities that populate our language is paramount. These entities, ranging from people to places, organizations, and events, provide a window into the core structure of the world around us. And when it comes to unlocking the true meaning of a text, identifying the key entities is essential.
So, what exactly are these entities?
Think of entities as the building blocks of our linguistic landscape. They’re the characters, the settings, the organizations, and the actions that drive the narrative of any text. Just as a house is built from a foundation of bricks, a text is built from a web of interconnected entities that shape its overall meaning and purpose.
But entities aren’t all created equal.
Some entities are more prominent than others, some more relevant to the context at hand, and some more elusive to identify. That’s why it’s important to establish a clear understanding of the types of entities that may be present in the text you’re analyzing.
Common Entity Types
The world of entities is a vast and varied one, but some of the most common types include:
- People: Individuals, such as authors, politicians, or historical figures.
- Places: Geographical locations, such as cities, countries, or landmarks.
- Organizations: Businesses, government agencies, or non-profit groups.
- Events: Occurrences, such as sporting events, concerts, or natural disasters.
- Products: Goods or services, such as cars, books, or software.
By understanding the different types of entities that might appear in your text, you’ll be better equipped to identify and extract them, unlocking the hidden gems of information that they hold.
**Establishing Scoring Criteria for Entity Retrieval**
In the realm of data analysis, identifying and extracting meaningful entities from text is a crucial step. To ensure accuracy and precision in this process, it’s essential to establish a robust scoring system that can evaluate entities based on their relevance and significance.
One commonly used scoring system assigns numerical values to different aspects of an entity, such as its frequency, context, and semantic similarity to the search query. Each factor is weighted differently, with higher weights given to more significant attributes. By combining these weighted scores, a comprehensive entity score is calculated.
In our specific search, we have defined a target score range of 8 to 10. This range represents entities that are highly relevant, frequent in the context, and semantically aligned with the query. By focusing on this narrow range, we aim to identify only the most suitable entities that meet our stringent criteria.
Searching for Elusive High-Scoring Entities
In our quest for valuable information, we set out to identify and extract the most relevant entities from a vast corpus of data. Armed with a meticulous scoring system, we carefully evaluated each entity, assigning scores that ranged from 1 to 10. Our goal was to uncover the crème de la crème—entities that soared above the rest with scores between 8 and 10.
We meticulously combed through the data, scrutinizing every nook and cranny for these exceptional entities. Yet to our astonishment, _not a single one _met our exacting criteria.
Undeterred, we delved into the possible causes for this puzzling absence. Perhaps the context we examined was too narrow or lacked sufficient depth, limiting the number of high-scoring entities available to us. Maybe the relevance of the entities themselves did not align with our specific search parameters. Or perhaps our scoring thresholds were simply too stringent.
Despite our exhaustive efforts and careful consideration, we were unable to pinpoint the reasons why we could not find any entities with scores between 8 and 10. This enigmatic discovery forced us to confront the implications and ponder the future course of our research.
Analyzing the Absence of High-Scoring Entities
Entity extraction is a crucial step in various natural language processing (NLP) applications. However, sometimes we may encounter scenarios where we fail to find entities that meet our desired scoring criteria. Understanding the potential reasons behind this absence can help us refine our retrieval strategies and improve our NLP models.
Factors Influencing Entity Absence:
-
Context Limitations: The context itself may not contain entities relevant to the specified score range. This can occur when the context is too narrow or focused on a specific aspect, excluding entities with higher scores.
-
Entity Relevance: The scoring criteria may not adequately capture the relevance of entities to the context. Entities that are inherently valuable but do not align perfectly with the scoring system may be missed.
-
Scoring Thresholds: The specified score range (8-10) may be too stringent for the given context. Entities with slightly lower scores but still valuable may be overlooked.
Consequences of Entity Absence:
-
Incomplete Analysis: High-scoring entities often provide valuable insights. Their absence can lead to an incomplete understanding of the context, potentially hindering decision-making or further analysis.
-
Missed Opportunities: Entities that closely align with the desired score range can offer significant benefits, such as identifying key individuals, organizations, or concepts. Failing to find them may result in missed opportunities for knowledge extraction and business intelligence.
Recommendations for Future Retrieval:
-
Re-evaluate Context: Examine the context thoroughly to assess if it provides sufficient depth and relevance for the desired entity types. If necessary, expand the context to include additional information.
-
Refine Scoring Criteria: Revisit the scoring system and consider adjusting weights or incorporating additional factors to better reflect entity relevance and significance.
-
Explore Different Retrieval Techniques: Utilize alternative search algorithms or NLP techniques that may be better suited for the specific context and entity types being targeted.
Implications of Finding No Matching Entities: A Puzzle to Unravel
The Search for Elusive Entities
In the realm of entity retrieval, our quest to uncover high-scoring entities often leads us on a winding path. Imagine our surprise when, despite our diligent efforts, we come up empty-handed—no entities meet our stringent criteria. This discovery can leave us scratching our heads, wondering, “What went wrong?”
Consequences of a Fruitless Search
The absence of high-scoring entities can have far-reaching implications. First and foremost, it hinders our ability to gain a deep understanding of the context we’re investigating. Entities serve as building blocks, anchoring our knowledge and providing essential context. Without them, our understanding remains incomplete and fragmented.
Moreover, the lack of high-scoring entities can invalidate our initial assumptions about the presence of certain key concepts or themes. This can lead to a misinterpretation of the context and potentially flawed conclusions.
Implications for Further Research and Analysis
The absence of matching entities also prompts us to re-evaluate our research strategy. It may necessitate expanding our search parameters, refining our scoring criteria, or delving deeper into the context to uncover hidden patterns or connections.
Furthermore, it can spark an exploration of alternative perspectives, challenging our preconceived notions and opening up new avenues of inquiry. The absence of high-scoring entities can act as a catalyst for creative thinking and the generation of innovative hypotheses.
Recommendations for Future Retrieval
To avoid similar disappointments in future entity retrieval endeavors, we recommend considering the following strategies:
- Refine Scoring Criteria: Re-evaluate the scoring system and thresholds to ensure they are appropriate for the context and research objectives.
- Expand Search Techniques: Explore alternative search algorithms and techniques to broaden the pool of potential entities.
- Enhance Context Analysis: Conduct a thorough analysis of the context to identify hidden relationships and dependencies that may have been overlooked initially.
By embracing these recommendations, we can increase the likelihood of finding meaningful entities and gaining a more comprehensive understanding of the data we seek to explore.
Recommendations for Future Entity Retrieval
Enhancing the Scoring Matrix
For future retrieval efforts, consider revisiting the scoring criteria to calibrate its sensitivity. Adjust the scoring thresholds to allow for a broader range of entities that meet the desired quality parameters. This fine-tuning can increase the likelihood of identifying entities that align with the intended score range.
Expanding Search Strategies
Broaden the search techniques employed to uncover hidden or elusive entities. Explore alternative algorithms or data sources that may offer complementary insights into the context. By casting a wider net, you increase the chances of discovering entities that might have been overlooked by the initial search parameters.
Refining Contextual Analysis
Delve deeper into the context to gain a more comprehensive understanding of the underlying concepts and relationships. Investigate additional sources of information to enrich the context and provide a more robust foundation for entity retrieval. By thoroughly examining the context, you can identify hidden patterns and uncover entities that are deeply interconnected with the topic.
Additional Tips for Enhanced Entity Retrieval
- Utilize natural language processing (NLP) tools to extract entities from unstructured text and improve search accuracy.
- Leverage machine learning algorithms to automate entity recognition and classification.
- Collaborate with domain experts to provide valuable insights and refine the retrieval process.
- Continuously evaluate and refine the retrieval pipeline to optimize performance and ensure consistent results.