Master Entity Extraction: Enhance Nlp Accuracy For Business Success
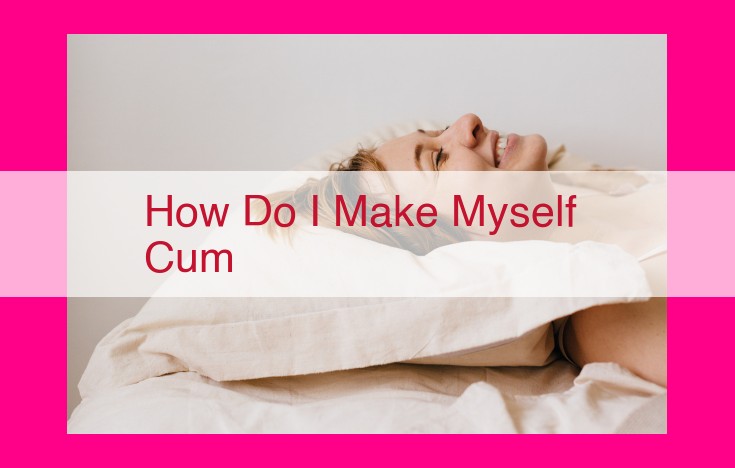
Understanding entity extraction, a crucial NLP technique for identifying and scoring entities in text, helps improve accuracy in extracting high-score entities. It addresses challenges, best practices, and applications in various industries. Despite limitations, ongoing research explores advancements in named entity recognition (NER) systems to enhance the quality and accuracy of entity extraction.
Unveiling the Secrets of Entity Extraction: Understanding the Art of Natural Language Comprehension
In the realm of natural language processing, there lies a remarkable technique known as entity extraction, an indispensable tool that allows us to unlock the hidden treasures of textual data. This sophisticated process enables computer systems to identify and comprehend the key entities mentioned within a body of text, shedding light on the underlying meaning and relationships within written language.
Named entity recognition (NER), a cornerstone of entity extraction, plays a pivotal role in this endeavor. NER systems are meticulously designed to pinpoint and categorize specific types of entities, such as names of people, organizations, locations, and dates. Through meticulous analysis of text, these systems recognize patterns and linguistic cues to discern the true nature of entities, transforming raw text into a structured and meaningful representation.
By identifying these entities, we gain a deeper understanding of the context and semantics of text. This knowledge unlocks a myriad of possibilities, empowering us to:
- Craft intelligent search engines that can navigate vast amounts of text with ease.
- Develop targeted marketing campaigns by extracting relevant information about customer profiles.
- Power virtual assistants with the ability to comprehend and respond to natural language queries.
The applications of entity extraction are as diverse as the texts themselves, extending across industries and disciplines, from finance and healthcare to social media and customer service.
Scoring Entities
In the realm of natural language processing, entity extraction is not merely about identifying and extracting entities from text. To truly harness the power of extracted entities, we must go a step further and score them based on their relevance or significance.
Scoring entities allows us to prioritize and focus on the most important information within a document. By assigning a numerical value to each entity, we can create a hierarchical ranking that guides our downstream tasks. This scoring process is crucial for applications like question answering, information retrieval, and machine translation, where the quality of the extracted entities directly impacts the accuracy and effectiveness of the system.
The factors that influence entity scoring are multifaceted. One key aspect is entity type. Certain types of entities, such as proper nouns and specific locations, typically carry more weight than common nouns or generic terms. For example, in a document about the history of artificial intelligence, the entity “Alan Turing” would likely receive a higher score than the entity “computer”.
Contextual relevance also plays a significant role. Entities that are directly related to the main topic or query of interest should be scored higher. In a biomedical research paper, for instance, entities related to specific diseases or treatments would be more relevant than entities related to general medical concepts.
Finally, entity salience influences its score. This refers to the prominence or visibility of an entity within the text. Entities that appear in multiple sentences or are mentioned in a prominent position, such as the title or abstract, tend to be more salient and thus receive a higher score.
Challenges in Extracting Entities with High Scores
Understanding the Difficulty
Extracting entities from text with high scores is no easy feat. The task faces numerous obstacles that often trip up even the most advanced NER systems.
Common Pitfalls and Error Sources
One significant challenge is ambiguity. Natural language is inherently ambiguous, with many words possessing multiple meanings depending on the context. This ambiguity can lead NER systems to misidentify or miss entities altogether.
Another common error source stems from incomplete or noisy data. Real-world datasets are often marred by missing values, typos, and other imperfections. These imperfections can confuse NER systems and make it difficult for them to extract accurate entities.
Additionally, the sheer volume of data can also pose a challenge. The vast amount of text available nowadays can overwhelm NER systems, making it difficult to process and extract entities in a timely and efficient manner.
Best Practices for High-Quality Extraction
Despite the challenges, there are best practices that can be employed to improve entity extraction accuracy. These include:
- Feature engineering: Extracting relevant features from the text can help NER systems better identify entities.
- Model fine-tuning: Retraining the NER model on a specific dataset can enhance its performance on the target domain.
- Ensemble methods: Combining multiple NER models can improve overall accuracy by leveraging their collective strengths.
Strategies for Enhancing Entity Extraction Accuracy
In the realm of natural language processing, extracting entities with high scores is a critical step towards unlocking the true potential of text data. However, this task is fraught with challenges, often resulting in imprecise or incomplete entity recognition. To overcome these obstacles, we must adopt a multifaceted approach that encompasses best practices, innovative techniques, and continuous refinement.
Best Practices for Enhanced Accuracy
Embracing industry-proven practices is a cornerstone of accurate entity extraction. Preprocessing the input text to remove noise and inconsistencies can significantly improve recognition accuracy. Tokenization breaks down the text into individual units, while lemmatization reduces words to their root form. Part-of-speech tagging identifies the grammatical role of each word, providing valuable context for entity identification.
Feature Engineering for Informative Extraction
Feature engineering plays a vital role in amplifying entity extraction capabilities. By adding rich features to the input data, we enhance the model’s understanding of the text. These features can include word embeddings, sentence structure, and external knowledge bases.
Model Fine-tuning for Precision
Fine-tuning pretrained models on task-specific datasets can dramatically improve entity recognition precision. This process involves adjusting the model’s parameters based on the target data distribution. By specializing the model to the specific domain, we enhance its ability to identify relevant entities with high accuracy.
Ensemble Methods for Robustness
Ensemble methods combine multiple models to create a single, more robust entity extraction system. Each model is trained on a different subset of the data or using different hyperparameters. By combining their predictions, we can mitigate the weaknesses of individual models and achieve higher overall accuracy.
These strategies, when implemented in a judicious manner, can significantly enhance the accuracy of entity extraction. By embracing best practices, leveraging feature engineering, fine-tuning models, and employing ensemble methods, we unlock the true potential of NLP for extracting meaningful insights from text data.
Extracting High-Value Entities: Unlocking the Power of Accurate Entity Recognition
In the realm of natural language processing, entity extraction takes center stage as an indispensable tool for identifying and extracting meaningful entities from unstructured text. Its role is not just to identify entities but also to assess their relevance and significance through scoring.
But extracting entities with high scores is no stroll in the park. Various challenges, such as lexical ambiguity, context dependency, and entity hierarchies, often rear their ugly heads. However, by implementing smart strategies like feature engineering, model fine-tuning, and ensemble methods, we can effectively mitigate these hurdles and enhance entity recognition accuracy.
So, what are the practical applications of this high-quality entity extraction? Let’s dive into some captivating use cases:
- Medicine: Extract patient demographics, symptoms, and medications from medical records to expedite diagnosis and treatment planning.
- Finance: Identify key financial entities (e.g., companies, currencies, amounts) from financial reports and news articles to facilitate informed investment decisions.
- Security: Uncover entities of interest in threat intelligence data, aiding in cybersecurity threat detection and prevention.
- Social Media: Analyze social media posts to extract entities such as sentiment, topics, and demographics, empowering brand monitoring and targeted marketing campaigns.
- Legal: Extract entities like names, dates, and locations from legal documents, streamlining discovery and document review processes.
In each of these industries, accurate entity extraction is paramount. It unveils valuable insights, automates tasks, and drives informed decision-making. By harnessing the power of high-quality entity extraction, we unlock a treasure trove of possibilities, transforming vast amounts of text into actionable data.
Limitations and Future Directions in Entity Extraction
Despite the advancements in entity extraction technology, there are still certain limitations that need to be addressed.
Limitations:
One challenge is dealing with ambiguity and context dependency. Entities often appear in complex contexts where their meaning can vary based on the surrounding words and phrases. This makes it difficult for NER systems to accurately identify and score entities in all situations.
Another limitation is the reliance on labeled data for training NER models. Labeled data is expensive and time-consuming to acquire, and it can be difficult to obtain sufficient data for all possible entity types and contexts. As a result, NER systems may perform poorly on unseen or rare entity types.
Future Directions in Entity Extraction Research
Researchers are actively exploring various approaches to overcome these limitations and improve the accuracy and efficiency of entity extraction.
Emerging Trends:
One promising area is the use of unsupervised and semi-supervised learning techniques. These methods can leverage large amounts of unlabeled text to train NER models, reducing the need for manual annotation.
Another trend is the development of contextualized NER models. These models take into account the surrounding context of entities, enabling them to better resolve ambiguity and identify entities more accurately.
Research Directions:
Future research in entity extraction will likely focus on the following areas:
- Improving entity scoring algorithms to better differentiate between relevant and irrelevant entities.
- Developing robust NER models that can handle complex and ambiguous text.
- Exploring new data sources and annotation methods to overcome the limitations of labeled data.
- Investigating the application of entity extraction to emerging domains, such as social media and scientific literature.
By addressing these limitations and pursuing exciting new research directions, the field of entity extraction is poised to make significant contributions to various industries and applications, unlocking valuable insights from unstructured text data.