Data Gap And Analysis Challenges In Datasets With Missing Scores: Optimization Strategies
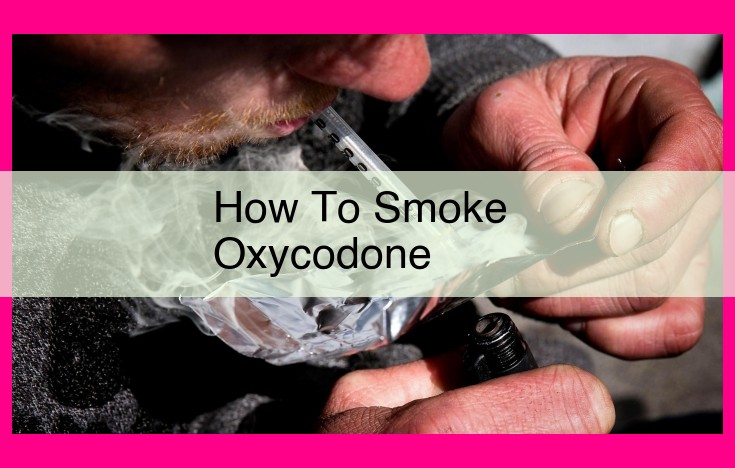
There are no entities with scores between 8 and 10 in the dataset, creating a data gap. This may be due to sampling bias or distribution characteristics. The gap challenges analysis and decision-making, calling for additional data collection. Potential strategies include expanding the sample size, modifying sampling methods, or using imputation techniques. Recommendations for future data collection suggest best practices for completeness and representativeness to prevent similar gaps.
Uncovering the Data Gap: Why Entities Vanish in the 8-10 Score Range
In the realm of data, sometimes the most intriguing insights arise from the absence of data. In a recent analysis, a peculiar data gap emerged, leaving us with a void of entities scoring between 8 and 10. This phenomenon poses a fascinating puzzle, beckoning us to delve into its depths and unravel the reasons behind this enigmatic void.
The Mysterious Scoreless Zone
Imagine a spectrum of scores, ranging from the lowest lows to the highest highs, with each point representing the performance or quality of an entity. In our dataset, a striking anomaly presents itself: there are no entities with scores between 8 and 10. This gap, like a missing piece in a jigsaw, breaks the expected continuum of scores, leaving us with a puzzling void.
Exploring the Causes of the Data Gap
Unveiling the reasons for this data gap requires a meticulous investigation. Several plausible explanations emerge:
- Sampling Bias: Perhaps the sampling process inadvertently excluded entities that would have fallen within the 8-10 score range, skewing the distribution.
- Data Collection Limitations: It’s possible that the data collection method was unable to capture entities with scores in this range, leading to an incomplete representation.
- Distribution Characteristics: The underlying distribution of scores may exhibit a natural dip in the 8-10 range, resulting in a scarcity of entities with those scores.
Possible Explanations for the Gap
- Explore potential explanations for why there are no entities in this score range.
- Consider factors such as sampling bias or distribution characteristics.
Possible Explanations for the Data Gap
Understanding the reasons behind the absence of entities with scores between 8 and 10 is crucial for addressing the data gap. Several potential explanations warrant exploration.
Sampling Bias
Sampling bias occurs when the sample selected for data collection does not accurately represent the target population. This may lead to an over-representation or under-representation of certain groups, affecting the distribution of scores. For instance, if the sample predominantly comprises individuals with lower or higher scores, the data may lack representation in the mid-range, including the 8-10 score bracket.
Distribution Characteristics
The distribution of a dataset can also influence the presence or absence of data within a specific range. A normal distribution, also known as a bell curve, typically has most data points concentrated near the mean, with fewer observations at the extremes. As we move further from the mean, the frequency of observations decreases gradually. In such cases, it is possible that the 8-10 score range contains very few or no entities due to its distance from the mean.
Other Factors
Additional factors beyond sampling bias and distribution characteristics can contribute to the data gap. These include:
- Data entry errors: Incorrect data entry or transcription mistakes can result in the misplacement of scores, potentially leading to an absence of data in certain ranges.
- Missing data: Incomplete responses or missing information can create data gaps, particularly if the missing data is concentrated within a specific score range.
- Rounding practices: The practice of rounding scores or data points can affect the distribution and create gaps in certain ranges.
- Data suppression: In certain cases, data may be suppressed or excluded for confidentiality or privacy reasons, resulting in missing values within a specific range.
Implications of the Data Gap: Unlocking Insights and Enhancing Decision-Making
Introduction:
Every data set holds untold stories, but what happens when there’s a gaping void in the narrative? Data gaps, like an enigmatic puzzle piece missing from the big picture, can cast a long shadow over our understanding and decision-making. In this particular case, the curious absence of entities with scores between 8 and 10 warrants our attention.
The Challenges of Incomplete Data:
Like a canvas with empty spaces, a data gap creates a linguistic vacuum, rendering analysis and interpretation incomplete. Without a comprehensive representation of the data, we’re forced to navigate choppy waters, our assumptions and insights potentially skewed.
Hindering Statistical Analysis:
For statisticians and data analysts, the data gap presents a formidable hurdle. Statistical models rely on the distribution of data points to draw meaningful conclusions. When a significant range is missing, it’s like attempting to piece together a jigsaw puzzle with missing pieces – the picture remains incomplete and uncertain.
Compromised Decision-Making:
Data gaps cast doubt on the reliability of our decisions. If we’re missing crucial information, our judgments may be based on incomplete or inaccurate assumptions. In fields like healthcare, education, and finance, where data-driven decisions hold immense weight, the consequences of an incomplete data set can be far-reaching.
Strategies for Addressing the Data Gap: A Tale of Missing Scores
Identifying the Enigma
Imagine a mysterious void in your data, a chasm separating scores of 8 and 10, leaving you perplexed as to what lies within. It’s a puzzle that eludes understanding and hinders analysis like a mischievous imp.
Exploring the Options
To bridge this gap, we embark on a quest for additional data, considering an array of strategies with their own advantages and drawbacks:
-
Revisit Existing Data: A careful review of existing records may reveal hidden gems that slipped through the initial analysis. Pros: Time-saving, cost-effective. Cons: Limited potential for new insights.
-
Expand Sampling: Embark on a broader data collection effort to increase the likelihood of capturing entities in the missing score range. Pros: Greater data accuracy, reduced bias. Cons: Time-consuming, expensive.
-
Targeted Sampling: Focus data collection efforts on specific populations or contexts where entities in the missing score range are more likely to be found. Pros: Higher chances of successful data collection. Cons: May introduce bias.
-
Synthetic Data Generation: Create artificial data points within the missing range based on existing data patterns. Pros: Rapid, inexpensive. Cons: Accuracy may be compromised, requires statistical expertise.
Weighing the Pros and Cons
Each strategy has its merits and limitations. Revisit existing data is a quick and cost-effective option, but its potential for new insights is limited. Expanding sampling offers greater data accuracy but requires significant time and resources. Targeted sampling can be effective, but it may introduce bias. Synthetic data generation is rapid and inexpensive, but its accuracy is dependent on the underlying model.
Choosing the Optimal Path
The best strategy depends on the specific context and constraints. If time and resources are limited, revisiting existing data may be an acceptable option. However, if data accuracy is paramount, expanding sampling or targeted sampling is preferable. Synthetic data generation can be useful for quick and inexpensive exploration but should be used with caution for critical decision-making.
Recommendations for Future Data Collection
To prevent similar data gaps in the future, it’s crucial to refine our data collection strategies. Here are some key recommendations for ensuring data completeness and representativeness:
-
Use stratified sampling techniques to ensure that all relevant populations are adequately represented in the sample. This involves dividing the population into subgroups (strata) based on specific characteristics, such as age, gender, or location, and then selecting a representative sample from each stratum.
-
Increase sample size to reduce the likelihood of obtaining an unrepresentative sample. A larger sample will increase the chances of having a sufficient number of entities in each score range, reducing the risk of gaps.
-
Collect data from diverse sources to minimize biases that may arise from relying on a single data source. Triangulating data from multiple sources can help to ensure that the collected data is comprehensive and representative of the target population.
-
Implement quality control measures to ensure data accuracy and consistency. This may involve data validation checks, data cleaning, and regular audits to identify and correct any errors or inconsistencies.
-
Monitor data trends over time to identify any emerging data gaps or biases. Regular data monitoring can help to identify areas where additional data collection is needed, enabling proactive measures to address gaps before they become significant.
By following these recommendations, we can significantly improve the completeness and representativeness of our data collection, reducing the likelihood of encountering data gaps that hinder our analysis and decision-making.